The tool identified patients with high-risk disease, which normally goes unrecognised by traditional diagnostics.
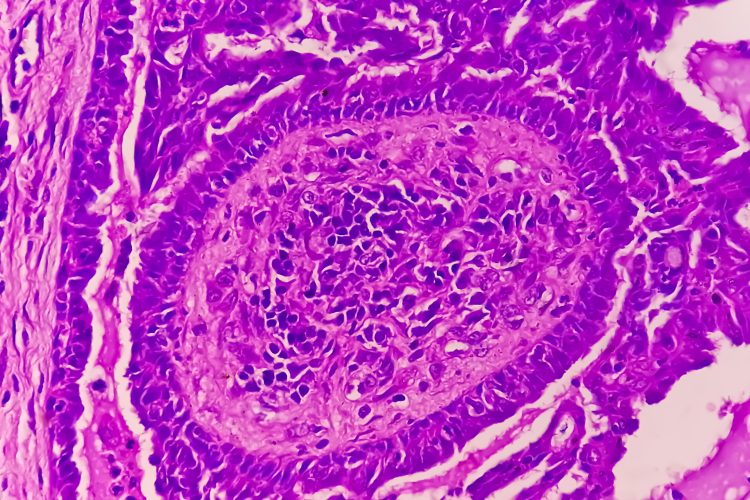
A distinct subset of endometrial cancer that puts patients at a much higher risk of recurrence and death has been determined by scientists at the University of British Columbia (UBC) using artificial intelligence (AI). Their discovery could improve treatment outcomes by aiding the identification of those with high-risk disease, which would typically go unrecognised by traditional pathology and molecular diagnostics.
Dr Jessica McAlpine, professor and Dr Chew Wei Chair in Gynaecologic Oncology at UBC, and surgeon-scientist at BC Cancer and Vancouver General Hospital, explained: “Endometrial cancer is a diverse disease, with some patients much more likely to see their cancer return than others…It’s so important that patients with high-risk disease are identified so we can intervene and hopefully prevent recurrence. This AI-based approach will help ensure no patient misses an opportunity for potentially lifesaving interventions.”
This study builds on research conducted by Dr McAlpine and her colleagues at British Columbia’s Gynecologic Cancer Initiative. In 2013, they found that endometrial cancer can be classified into four subtypes based on the molecular characteristics of cancerous cells, with each posing a different level of risk. They then developed a molecular diagnostic tool, named ProMiSE, which can accurately distinguish the subtypes. Currently, ProMiSE is used across B.C. and other parts of Canada, as well as internationally, to inform treatment decisions.
However, the most common molecular subtype, comprising around 50 percent of all cases, is a catch-all category for endometrial cancers without distinguishable molecular features. To overcome this issue, Dr McAlpine collaborated with Dr Ali Bashashati, assistant professor of biomedical engineering and pathology and laboratory medicine at UBC, to develop a deep learning AI model. The model was trained to differentiate between different subtypes. Following analysis of 2,318 cancer tissue images, a new subgroup that showed distinctly lower survival rates was found.
Moving forwards, the researchers are now investigating how to integrate the tool into clinical practice with traditional molecular and pathology diagnostics. The AI is cost efficient and easy to deploy across geographies, as it analyses images that are regularly gathered by pathologists, even at smaller sites in rural and remote areas. It may also enable patients to stay in their community for less intensive surgery and ensure those that require treatment at a larger centre can go.
Dr Bashashati concluded: “What is really compelling to us is the opportunity for greater equity and access…The AI doesn’t care if you’re in a large urban centre or rural community, it would just be available, so our hope is that this could really transform how we diagnose and treat endometrial cancer for patients everywhere.”
This study was published in Nature Communications.