Patient population
To explore the correction for collider bias utilising external data, data from Covid-19 positive patients admitted to Guy’s and St. Thomas’ NHS Foundation Trust (GSTT) between 20th February 2020 and 24th May 2021 was used. Patients were restricted to adults (18+) admitted from the community after 28th January 2020 (the date of the first known Covid-19 case in the UK) with a positive Covid-19 test within 28 days of admission who had known age, sex, Index of Multiple Deprivation (IMD). IMD is a relative measure of deprivation for small regional areas in the UK based on 7 domains of deprivation identified by the patient’s current address [30]. Medical history for these patients was collated from 6 linked databased and categorised as presence/absence of cardiovascular disease (stroke, transient ischaemic attack, atrial fibrillation, congestive heart failure, ischaemic heart disease, valve disease, peripheral artery disease or atherosclerotic disease), diabetes mellitus, chronic kidney disease, chronic liver disease, and chronic obstructive pulmonary disease/emphysema. The application of “do not attempt resuscitation” (DNR) orders was also extracted along with the date of application. These covariates were chosen for inclusion in this analysis based on known association with Covid-19 outcomes and known links to ethnicity. Ethnicity was categorized as White (British, European, Other), Black (African, Caribbean), Asian (South, South-East, and East Asian), Mixed/Other (Middle Eastern, South American, and Mixed) and Unknown (or not reported). The cohort characteristics are shown in the appendix (Appendix Page 8, Appendix Table 1 & Appendix Fig. 2).
Patients were categorised into two Covid-19 waves based on admission date for analysis purposes. Admission was designated as belonging to the first (until 31st August 2020) or second (from 1st September 2020) wave of Covid-19 matching timepoints used to analyse the OPENSAFELY cohort. This categorisation, along with ethnicity and survival status, informed the IPW (described below) given to each patient.
Statistical modelling
Time to death was analysed using Cox proportional hazards models with discharge as a competing event and robust standard errors. The association of ethnicity with death was assessed using three model iterations: (1) unadjusted (with no additional covariates), (2) adjusted for all described covariates, (3) adjusted for covariates and using IPW based on the probabilities derived below.
Part 1: Estimating the probability of Covid-19 associated hospitalisation in the first wave from national data
Estimating the necessary probabilities for IPW (following the principles laid out by Thompson and Arah [25]) requires an estimation of the risk of hospitalisation overall and estimation of the risk ratios (RRs) describing the relationship between hospitalisation and different ethnic groups stratified by survival status. These can be derived from a generalised linear model (GLM) for the probability of hospitalisation (Eq. 1).
(1)
Importantly the relationship between ethnicity and Covid-19 outcomes – including hospitalisation and death – has been reported for a national cohort in the UK. Mathur et al. [20] completed an analysis of the OPENSAFELY platform which holds electronic health record data from individuals registered with English primary care practices allowing an ideal reference point for estimating the probability of hospitalisation in different ethnic groups. Summary-level data from this publication included the number of adults from 16 different ethnic categories who were hospitalised with Covid-19 or experienced death associated with Covid-19 across the first (1st February to 3rd August 2020) and partial-second (1st September to 31st December 2020) wave of Covid-19.
Cross-tabulation of hospitalisation and survival status was not provided by Mathur et al. and so it was assumed initially that 90% of individuals who died were hospitalised prior to death (regardless of ethnicity). This assumption is based on the understanding that those who died had more severe disease and were more likely to be hospitalised along with an expectation not all deaths would occur in hospital. Based on this assumption and the summarised data estimates for the parameters in Eq. 1 could be obtained (Table 1) allowing the probability of hospitalisation with Covid-19 to be modelled for each ethnic group stratified by survival status. The inverse of these probabilities formed the sampling weights used as IPW allowing the conditional causal effect effect of ethnicity to be estimated as applied to a pseudo-population more representative of that in the community.
Part 2: Sensitivity analysis examining the effect of changes in estimated probabilities to match the local population
As the IPW used in this analysis is externally obtained data, it is important to validate it’s use by examining whether misspecification of the model parameters describing the association between ethnicity/death and hospitalisation would alter the obtained results.
Additional sources of data describing the risk of hospitalisation for specific ethnicities and survival status can be obtained from a combination of NHS and Office of National Statistics (ONS) sources [21,22,23,24]. Examination of these data may identify the extent to which the probability of hospitalisation as calculated for the national cohort examined by OPENSAFELY [20] differed for a local London-based (GSTT) cohort and so the likely degree of misspecification that is present for the model parameters in Eq. 1. Two comparisons were made: one utilizing data specific to the GSTT catchment area (i.e. NHS reported cases for GSTT; ONS estimates for Lambeth, Lewisham and Southwark) (Table 2) and one using data relevant to the Greater London area (Appendix Table 2).
These comparisons found comparable risks of hospitalisation overall in the OPENSAFELY cohort compared to that expected for London (informing misspecification of \({\widehat{\gamma }}_{0}\)). the assumption that 90% of patients who died from Covid-19 were hospitalised (informing \({\widehat{\gamma }}_{1}\), RR comparing those who died vs., those who survived) was shown to be incorrect. ONS provided data on the place of Covid-19 associated death estimated that only 72–82% of individuals in London died in hospital.
Rates of hospitalisation were not stratified by ethnicity. To allow the degree of misspecification to be explored for \({\widehat{\varvec{\lambda }}}_{\varvec{a}}\) (RRs comparing ethnic groups in those who didn’t die) to be determined, the risk of hospitalisation for each ethnicity was estimated from the GSTT cohort residing in the catchment area divided by the ONS population estimates for the same area. The relative risk of hospitalisation in minority ethnicities compared to White ethnicity was larger for the GSTT cohort than that calculated using the OPENSAFELY data. These risks were comparable to that found for an independent East London cohort (ETHICAL [13]) and is likely due to the high prevalence of these ethnicities in London compared to the national population and the increased risk of infection these groups face due to societal, cultural, and occupational pressures [31,32,33].
Ethnicity-specific data was not available for the place of Covid-19 associated death. As such, applying degrees of misspecification to \({\widehat{\varvec{\lambda }}}_{\varvec{b}}\) (parameter describing the effect of interaction between ethnicity and death on hospitalisation) was based on conjecture. News articles suggested hesitancy towards hospital care by some ethnic groups in the first wave of the pandemic [34]. Therefore \({\widehat{\varvec{\lambda }}}_{\varvec{b}}\) was adjusted to reduce the probability of hospitalisation in those from minority ethnicities who died.
The differences in log risk/RRs between the OPENSAFELY analysis and GSTT specific data (Table 2) provided guides for the level of misspecification which should be applied to each fitted parameter in Eq. 1. Comparison between the OPENSAFELY analysis and data for the Greater London area (Appendix Table 2) often suggested more extreme differences than that suggested when comparing the OPENSAFELY analysis to GSTT specific data (usually in the same direction). As a result, three levels of misspecification were applied to each parameter (Table 3) allowing for differing degrees of misspecification. These were: approximation of the log risk/RRs calculated in Table 2 (called 100%), a milder degree of misspecification (called 50%), and a more extreme degree of misspecification akin to that seen for the Greater London area demonstrated in Appendix Table 2 (called 200%). Each set of probabilities obtained were checked for appropriate expected changes which remained within the range of {0–1} prior to use. This resulted in some adjustments to the approximate values described here.
The aim was to examine the misspecification of each parameter separately. However, adjustment of \({\widehat{\varvec{\lambda }}}_{\varvec{a}}\) would have resulted in the probability of hospitalisation in those who died to be above 1. To allow the suggested misspecification of \({\widehat{\varvec{\lambda }}}_{\varvec{a}}\) a 100% misspecification of \({\widehat{\gamma }}_{1}\) (value of -0.15) was also applied. This meant that the linear predictor (originally from Eq. 1) to which misspecification was applied became:
$${\eta }_{i}={\widehat{\gamma }}_{0}+({\widehat{\gamma }}_{1}-0.15){ death}_{\varvec{i}}+{\widehat{\varvec{\lambda }}}_{\varvec{a}}{\varvec{e}}_{\varvec{i}}+{{\widehat{\varvec{\lambda }}}_{\varvec{b}}{\varvec{e}}_{\varvec{i}}death}_{\varvec{i}}$$
After each level of misspecification was applied to the fitted model, the probability of hospitalisation for each ethnicity/survival status combination was recalculated, applied to the GSTT cohort and used as weights in the weighted analyses as per the original IPW analysis.
Part 3: Addition of covariates into the model equation
The methodology suggested by Thompson and Arah [25] allows the inclusion of covariates in the model determining probability of inclusion within a dataset. For a Covid-19 based analysis, one factor to consider is the wave of Covid-19. Research – including the OPENSAFELY analysis – indicates a time dependence for the risk of hospitalisation and mortality within different ethnic subgroups [11, 20, 27]. Therefore, accounting for this chance in risk when including data from multiple Covid-19 waves in a single IPW weighted analysis is vital.
As Mathur et al.’s analysis of the OPENSAFELY cohort includes separate data from the first (1st February to 3rd August 2020) and partial-second (1st September to 31st December 2020) wave of Covid-19 this same external data set can be used to develop IPWs from a model estimating the risk of hospitalisation based on patient ethnicity, survival status, and Covid-19 wave at admission. Adapting the initial model (Eq. 1), the risk can be calculated from a new GLM with the added elements indicated in blue (Eq. 2).
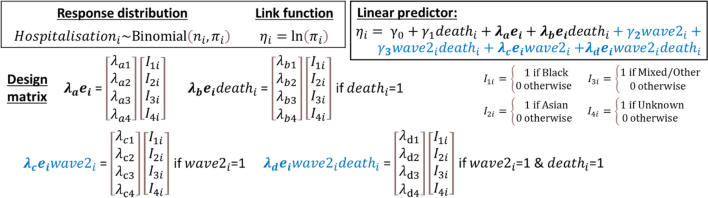
(2)
Following the same principles as described in the above sections, summarised data from the OPENSAFELY analysis across the first two waves of Covid-19 was applied to the model in Eq. 2 (Table 4). The calculated risks of hospitalisation for each ethnicity/wave/survival status group were inverted to create IPWs to apply to a weighted version of a Cox proportional hazards model with discharge as a competing risk. Degrees of misspecification likely to apply to the new model parameters in Eq. 2 were determined by comparing the OPENSAFELY data to other external sources (Appendix Tables 2 and 3). These model parameters were adjusted based on these comparisons (Table 3) and applied as part of a sensitivity analysis examining the effect of misspecification on the obtained results.