14 Jun Coronary Artery Disease: Rare Genetic Variants Using Machine Learning Model
MedicalResearch.com Interview with:
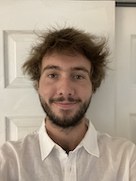
Ben Petrazzini
Ben Omega Petrazzini, B.Sc.
Associate Bioinformatician
Ron Do Laboratory
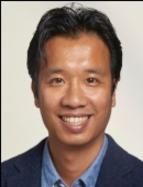
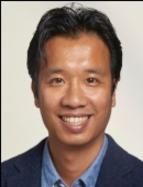
Dr. Ron Do
Ron Do, Ph.D.
Professor, Department of Genetics and Genomic Sciences
Director, Center for Genomic Data Analytics
Associate Director in Academic Affairs, The Charles Bronfman Institute for Personalized Medicine
Charles Bronfman Professor in Personalized Medicine
Icahn School of Medicine at Mount Sinai
MedicalResearch.com: What is the background for this study?
Response: Rare coding variants directly affect protein function and can inform the role of a gene in disease.
Discovery of rare coding variant associations for coronary artery disease (CAD) to date have only had limited success. Genetic studies typically use standard phenotyping approaches to classify cases versus controls for CAD. However, this phenotyping approach doesn’t capture disease progression or severity in individuals.
We recently introduced an in-silico score for CAD (ISCAD) that tracks CAD progression, severity, underdiagnosis and mortality (Forrest et al. The Lancet, 2023, PMID 36563696). ISCAD was built using a machine learning model trained on clinical data from electronic health records (EHR). Importantly, ISCAD is a quantitative score that measures CAD on a spectrum. The quantitative nature of the score provides an opportunity to discover additional rare coding variant associations that may not have been detected with the standard case-control phenotyping approach.
Here in this study, we performed a large-scale rare variant association study in the exome sequences of 604,915 individuals for ISCAD, a machine learning-based score for CAD.
MedicalResearch.com: Is this a model that can be incorporated into existing genetic profiles or medical records?
Response: ISCAD uses diagnostic codes, medication prescriptions, laboratory test results and vital sign measurements from EHR to compute its prediction of CAD diagnosis.
As such, ISCAD can be calculated in any biobank with EHR data. Once ISCAD is calculated, genetic association analyses can be performed with ISCAD.
MedicalResearch.com: What should readers take away from your report?
Response: The study identified rare and ultra-rare coding variants in 17 genes associated with ISCAD. Several of these genes are known and found to be associated with lipid and lipoprotein traits. However, other genes were new. Further investigation into these genes will provide biological and mechanistic insights into their role on disease.
MedicalResearch.com: What recommendations do you have for future research as a results of this study?
Response: This study suggests that machine learning-based scores is a complementary phenotyping approach for genetic association studies. Future research includes more functional characterization of the new genes discovered in this study and conducting rare variant association testing with ISCAD in additional EHR-linked biobanks of diverse ancestries.
No disclosures
Citation:
Petrazzini, B.O., Forrest, I.S., Rocheleau, G. et al. Exome sequence analysis identifies rare coding variants associated with a machine learning-based marker for coronary artery disease. Nat Genet (2024). https://doi.org/10.1038/s41588-024-01791-x
The information on MedicalResearch.com is provided for educational purposes only, and is in no way intended to diagnose, cure, or treat any medical or other condition.
Some links may be sponsored. Products are not endorsed.
Always seek the advice of your physician or other qualified health and ask your doctor any questions you may have regarding a medical condition. In addition to all other limitations and disclaimers in this agreement, service provider and its third party providers disclaim any liability or loss in connection with the content provided on this website.
Last Updated on June 14, 2024 by Marie Benz MD FAAD
119