(Image: S Sioni/CMS-PHO-EVENTS-2021-004-2/M Rayner)
One of the main goals of the LHC experiments is to look for signs of new particles, which could explain many of the unsolved mysteries in physics. Often, searches for new physics are designed to look for one specific type of new particle at a time, using theoretical predictions as a guide. But what about searching for unpredicted – and unexpected – new particles? Trawling through the billions of collisions that occur in the LHC experiments without knowing exactly what to look for would be a mammoth task for physicists. So, instead of sifting through the data and looking for anomalies, the ATLAS and CMS collaborations are letting artificial intelligence (AI) do the job.
At the Rencontres de Moriond conference on 26 March, physicists from the CMS collaboration presented the latest results obtained by using various machine learning techniques to search for pairs of “jets”. These jets are collimated sprays of particles originating from strongly interacting quarks and gluons. They are particularly difficult to analyse, but they could be hiding new physics.
Researchers at ATLAS and CMS use several strategies to train AI algorithms in their searches for jets. By studying the shape of their complex energy signatures, scientists can determine what particle created the jet. Using real collision data, physicists at both experiments are training their AI to recognise the characteristics of jets originating from known particles. The AI is then able to differentiate between these jets and atypical jet signatures, which potentially indicate new interactions. These would show up as an accumulation of atypical jets in the data set.
Another method involves instructing the AI algorithm to consider the entire collision event and look for anomalous features in the different particles detected. These anomalous features may indicate the presence of new particles. This technique was demonstrated in a paper released by ATLAS in July 2023, which featured one of the first uses of unsupervised machine learning in an LHC result. At CMS, a different approach involves physicists creating simulated examples of potential new signals and then tasking the AI with identifying collisions in the real data that are different to regular jets but resemble the simulation.
In the latest results presented by CMS, each AI training method exhibited varying sensitivities to different types of new particles, and no single algorithm proved to be the best. The CMS team was able to limit the rate of production of several different types of particles that produce anomalous jets. They were also able to show that the AI-led algorithms significantly enhanced the sensitivity to a wide range of particle signatures in comparison to traditional techniques.
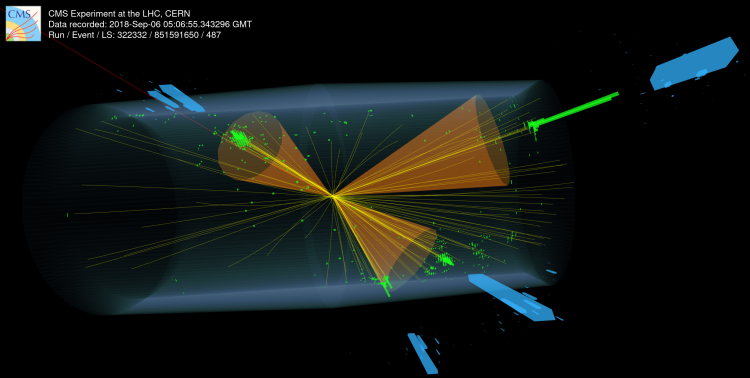
These results show how machine learning is revolutionising the search for new physics. “We already have ideas about how to further improve the algorithms and apply them to different parts of the data to search for several kinds of particles,” says Oz Amram, from the CMS analysis team.